This post is about the magic constant 0x5f3759df
and an extremely neat hack, fast inverse square root, which is where the constant comes from.
Meet the inverse square root hack:
float FastInvSqrt(float x) {
float xhalf = 0.5f * x;
int i = *(int*)&x; // evil floating point bit level hacking
i = 0x5f3759df - (i >> 1); // what the fuck?
x = *(float*)&i;
x = x*(1.5f-(xhalf*x*x));
return x;
}
What this code does is calculate, quickly, a good approximation for

It’s a fairly well-known function these days and first became so when it appeared in the source of Quake III Arena in 2005. It was originally attributed to John Carmack but turned out to have a long history before Quake going back through SGI and 3dfx to Ardent Computer in the mid 80s to the original author Greg Walsh. The concrete code above is an adapted version of the Quake code (that’s where the comments are from).
This post has a bit of fun with this hack. It describes how it works, how to generalize it to any power between -1 and 1, and sheds some new light on the math involved.
(It does contain a fair bit of math. You can think of the equations as notes – you don’t have to read them to get the gist of the post but you should if you want the full story and/or verify for yourself that what I’m saying is correct).
Why?
Why do you need to calculate the inverse of the square root – and need it so much that it’s worth implementing a crazy hack to make it fast? Because it’s part of a calculation you do all the time in 3D programming. In 3D graphics you use surface normals, 3-coordinate vectors of length 1, to express lighting and reflection. You use a lot of surface normals. And calculating them involves normalizing a lot of vectors. How do you normalize a vector? You find the length of the vector and then divide each of the coordinates with it. That is, you multiply each coordinate with
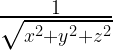
Calculating
is relatively cheap. Finding the square root and dividing by it is expensive. Enter FastInvSqrt
.
What does it do?
What does the function actually do to calculate its result? It has 4 main steps. First it reinterprets the bits of the floating-point input as an integer.
int i = *(int*)&x; // evil floating point bit level hack
It takes the resulting value and does integer arithmetic on it which produces an approximation of the value we’re looking for:
i = 0x5f3759df - (i >> 1); // what the fuck?
The result is not the approximation itself though, it is an integer which happens to be, if you reinterpret the bits as a floating point number, the approximation. So the code does the reverse of the conversion in step 1 to get back to floating point:
x = *(float*)&i;
And finally it runs a single iteration of Newton’s method to improve the approximation.
x = x*(1.5f-(xhalf*x*x));
This gives you an excellent approximation of the inverse square root of x. The last part, running Newton’s method, is relatively straightforward so I won’t spend more time on it. The key step is step 2: doing arithmetic on the raw floating-point number cast to an integer and getting a meaningful result back. That’s the part I’ll focus on.
What the fuck?
This section explains the math behind step 2. (The first part of the derivation below, up to the point of calculating the value of the constant, appears to have first been found by McEniry).
Before we can get to the juicy part I’ll just quickly run over how standard floating-point numbers are encoded. I’ll just go through the parts I need, for the full background wikipedia is your friend. A floating-point number has three parts: the sign, the exponent, and the mantissa. Here’s the bits of a single-precision (32-bit) one:
s e e e e e e e e m m m m m m m m m m m m m m m m m m m m m m m
The sign is the top bit, the exponent is the next 8 and the mantissa bottom 23. Since we’re going to be calculating the square root which is only defined for positive values I’m going to be assuming the sign is 0 from now on.
When viewing a floating-point number as just a bunch of bits the exponent and mantissa are just plain positive integers, nothing special about them. Let’s call them E and M (since we’ll be using them a lot). On the other hand, when we interpret the bits as a floating-point value we’ll view the mantissa as a value between 0 and 1, so all 0s means 0 and all 1s is a value very close to but slightly less than 1. And rather than use the exponent as a 8-bit unsigned integer we’ll subtract a bias, B, to make it a signed integer between -127 and 128. Let’s call the floating-point interpretation of those values e and m. In general I’ll follow McEniry and use upper-case letters for values that relate to the integer view and and lower-case for values that relate to the floating-point view.
Converting between the two views is straightforward:


For 32-bit floats L is 223 and B is 127. Given the values of e and m you calculate the floating-point number’s value like this:

and the value of the corresponding integer interpretation of the number is

Now we have almost all the bits and pieces I need to explain the hack so I’ll get started and we’ll pick up the last few pieces along the way. The value we want to calculate, given some input x, is the inverse square root or

For reasons that will soon become clear we’ll start off by taking the base 2 logarithm on both sides:

Since the values we’re working with are actually floating-point we can replace x and y with their floating-point components:

Ugh, logarithms. They’re such a hassle. Luckily we can get rid of them quite easily but first we’ll have to take a short break and talk about how they work.
On both sides of this equation we have a term that looks like this,

where v is between 0 and 1. It just so happens that for v between 0 and 1, this function is pretty close to a straight line:
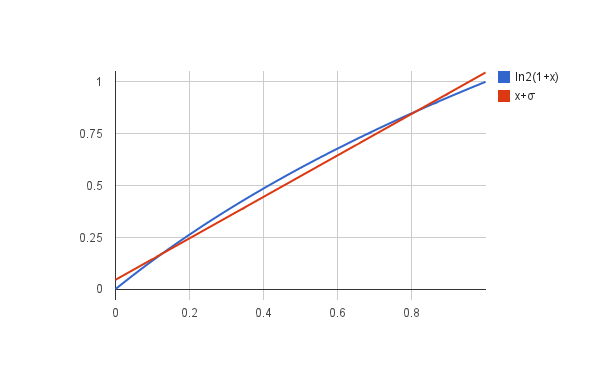
Or, in equation form:

Where σ is a constant we choose. It’s not a perfect match but we can adjust σ to make it pretty close. Using this we can turn the exact equation above that involved logarithms into an approximate one that is linear, which is much easier to work with:

Now we’re getting somewhere! At this point it’s convenient to stop working with the floating-point representation and use the definitions above to substitute the integer view of the exponent and mantissa:

If we shuffle these terms around a few steps we’ll get something that looks very familiar (the details are tedious, feel free to skip):



After this last step something interesting has happened: among the clutter we now have the value of the integer representations on either side of the equation:

In other words the integer representation of y is some constant minus half the integer representation of x. Or, in C code:
i = K - (i >> 1);
for some K. Looks very familiar right?
Now what remains is to find the constant. We already know what B and L are but we don’t have σ yet. Remember, σ is the adjustment we used to get the best approximation of the logarithm, so we have some freedom in picking it. I’ll pick the one that was used to produce the original implementation, 0.0450465. Using this value you get:

Want to guess what the hex representation of that value is? 0x5f3759df
. (As it should be of course, since I picked σ to get that value.) So the constant is not a bit pattern as you might think from the fact that it’s written in hex, it’s the result of a normal calculation rounded to an integer.
But as Knuth would say: so far we’ve only proven that this should work, we haven’t tested it. To give a sense for how accurate the approximation is here is a plot of it along with the accurate inverse square root:
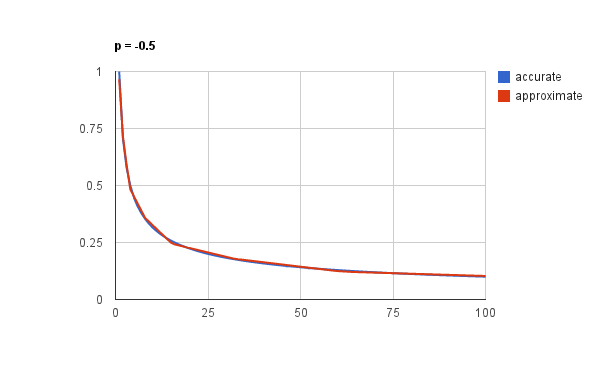
This is for values between 1 and 100. It’s pretty spot on right? And it should be – it’s not just magic, as the derivation above shows, it’s a computation that just happens to use the somewhat exotic but completely well-defined and meaningful operation of bit-casting between float and integer.
But wait there’s more!
Looking at the derivation of this operation tells you something more than just the value of the constant though. You will notice that the derivation hardly depends on the concrete value of any of the terms – they’re just constants that get shuffled around. This means that if we change them the derivation still holds.
First off, the calculation doesn’t care what L and B are. They’re given by the floating-point representation. This means that we can do the same trick for 64- and 128-bit floating-point numbers if we want, all we have to do is recalculate the constant which it the only part that depends on them.
Secondly it doesn’t care which value we pick for σ. The σ that minimizes the difference between the logarithm and x+σ may not, and indeed does not, give the most accurate approximation. That’s a combination of floating-point rounding and because of the Newton step. Picking σ is an interesting subject in itself and is covered by McEniry and Lomont.
Finally, it doesn’t depend on -1/2. That is, the exponent here happens to be -1/2 but the derivation works just as well for any other exponent between -1 and 1. If we call the exponent p (because e is taken) and do the same derivation with that instead of -1/2 we get:

Let’s try a few exponents. First off p=0.5, the normal non-inverse square root:


or in code form,
i = 0x1fbd1df5 + (i >> 1);
Does this work too? Sure does:
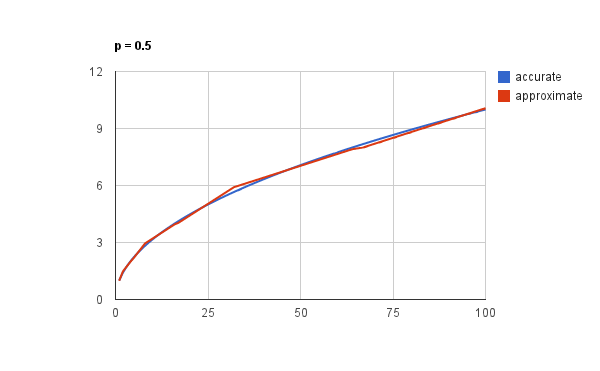
This may be a well-known method to approximate the square root but a cursory google and wikipedia search didn’t suggest that it was.
It even works with “odd” powers, like the cube root


which corresponds to:
i = (int) (0x2a517d3c + (0.333f * i));
Since this is an odd factor we can’t use shift instead of multiplication. Again the approximation is very close:
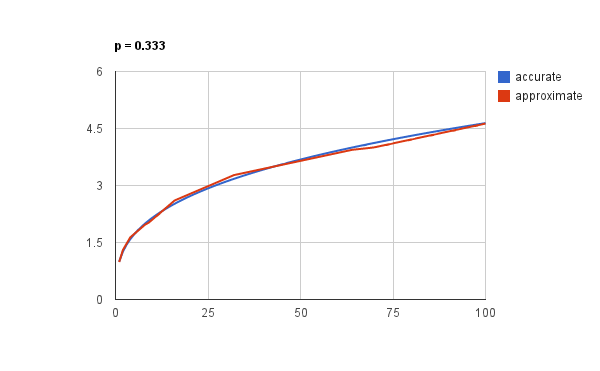
At this point you may have noticed that when changing the exponent we’re actually doing something pretty simple: just adjusting the constant by a linear factor and changing the factor that is multiplied onto the integer representation of the input. These are not expensive operations so it’s feasible to do them at runtime rather than pre-compute them. If we pre-multiply just the two other factors:

we can calculate the value without knowing the exponent in advance:
i = (1 - p) * 0x3f7a3bea + (p * i);
If you shuffle the terms around a bit you can even save one of multiplications:
i = 0x3f7a3bea + p * (i - 0x3f7a3bea);
This gives you the “magic” part of fast exponentiation for any exponent between -1 and 1; the one piece we now need to get a fast exponentiation function that works for all exponents and is as accurate as the original inverse square root function is to generalize the Newton approximation step. I haven’t looked into that so that’s for another blog post (most likely for someone other than me).
The expression above contains a new “magical” constant, 0x3f7a3bea
. But even if it’s in some sense “more magical” than the original constant, since that can be derived from it, it depends on an arbitrary choice of σ so it’s not universal in any way. I’ll call it Cσ and we’ll take a closer look at it in a second.
But first, one sanity check we can try with this formula is when p=0. For a p of zero the result should always be 1 since x0 is 1 independent of x. And indeed the second term falls away because it is multiplied by 0 and so we get simply:
i = 0x3f7a3bea;
Which is indeed constant – and interpreted as a floating-point value it’s 0.977477 also known as “almost 1” so the sanity check checks out. That tells us something else too: Cσ actually has a meaningful value when cast to a float. It’s 1; or very close to it.
That’s interesting. Let’s take a closer look. The integer representation of Cσ is

This is almost but not quite the shape of a floating-point number, the only problem is that we’re subtracting rather than adding the second term. That’s easy to fix though:

Now it looks exactly like the integer representation of a floating-point number. To see which we’ll first determine the exponent and mantissa and then calculate the value, cσ. This is the exponent:

and this is the mantissa:

So the floating-point value of the constant is (drumroll):

And indeed if you divide our original σ from earlier, 0.0450465, by 2 you get 0.02252325; subtract it from 1 you get 0.97747675 or our friend “almost 1” from a moment ago. That gives us a second way to view Cσ, as the integer representation of a floating-point number, and to calculate it in code:
float sigma = 0.0450465;
float c_sigma = 1 - (0.5f * sigma);
int C_sigma = *(*int)&c_sigma;
Note that for a fixed σ these are all constants and the compiler should be able to optimize this whole computation away. The result is 0x3f7a3beb
– not exactly 0x3f7a3bea
from before but just one bit away (the least significant one) which is to be expected for computations that involve floating-point numbers. Getting to the original constant, the title of this post, is a matter of multiplying the result by 1.5.
With that we’ve gotten close enough to the bottom to satisfy at least me that there is nothing magical going on here. For me the main lesson from this exercise is that bit-casting between integers and floats is not just a meaningless operation, it’s an exotic but very cheap numeric operation that can be useful in computations. And I expect there’s more uses of it out there waiting to be discovered.
(Update: I’ve added an appendix about the intuition behind the part where I shuffle around the floating-point terms and the integer terms appear.)